CBS is bidding farewell to its 'Murphy Brown' reboot and Damon Wayans Jr. led
'Happy Together,' with both shows ending after their initial 13-episode orders. The
'Murphy Brown' revival, once again starring Candice Bergen in the titular role, was always
intended to be a closed-ended order of 13 episodes. The multicamera comedy from
original creator Diane English, has underperformed in its prime Thursdays at 9:30 p.m.
slot and remains in consideration for a renewal. It will be replaced by fellow multicamera
comedy 'Fam' starting January 10. For its part, 'Happy Together' is CBS' only rookie
series to not earn an order for additional episodes. The multicamera family comedy
featured 'Happy Endings' star Damon Wayans Jr., who signed on with one of the largest
talent deals of the year, but failed to bring audiences to the table. The series will wrap its
run and clear way for Matt LeBlanc's 'Man With a Plan' starting Monday, Feb. 4 at
8:30 p.m. Meanwhile, 'Criminal Minds,' will go on a hiatus until a date to be determined in
2019. Sophomore drama 'SEAL Team' will take its time slot. Additionally, a return date for
the fourth season of 'Life in Pieces' will be announced at a later time as will premiere
dates for 'The Code' and 'The Red Line.' The news of the fate of 'Murphy Brown' and
'Happy Together' comes just a day after CBS announced that two new unscripted series
— 'The World's Best' and 'Million Dollar Mile' — would join a midseason roster that
includes returning favorites 'Survivor,' 'The Amazing Race' and 'Celebrity Big Brother.'
To read more on this story, head to THR.com.
For The Hollywood Reporter News, I'm Lyndsey Rodrigues.
For more infomation >> 'Murphy Brown' and 'Happy Together' to End as CBS Continues to Solidify Midseason Lineup | THR News - Duration: 1:30.-------------------------------------------
Overcoming Fear of Failure So You Can Succeed as an Entrepreneur - Duration: 6:48.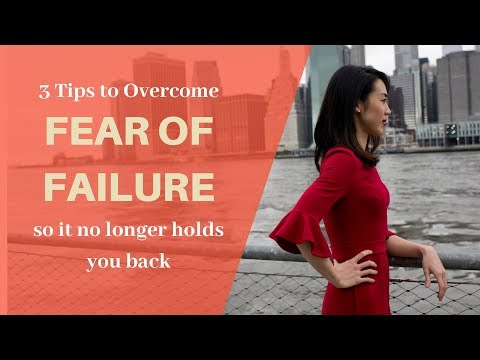
Failure.
That word can be so scary.
So how do you overcome fear of failure and not let it hold you back?
Hey there, this is Luisa Zhou and today I'm going to share 3 powerful tips to help you
eliminate that fear so that you can successfully achieve your business goals.
One more thing before we dive right in...Make sure you hit that subscribe button to get
notified when I share a new video every week on building your online business.
When I started my first online business, I was almost paralyzed by my fear of failure,
especially of putting myself out there and having everyone SEE me fail.
Despite all of that, I was able to overcome my fear and build that business to over 6-figures
BEFORE I left my job.
So, let's talk about how YOU can use my tips to conquer YOUR fear.
Secret #1 Stop seeing failure as an end point
When I was starting my first online business, every little failure and rejection felt like
a physical blow.
I remember one time, I submitted an article idea to a very popular website that you might
have heard of - Lifehacker - and I was so certain I was going to get accepted.
Every morning for weeks, I'd check my email and obsessively hit refresh, waiting for the
reply I knew was coming.
It never came.
And I remember thinking...I'm never going to succeed, because of this ONE rejection.
Thank goodness I didn't give up, because after that, I had about 50 other failures,
including about 2 months where I spoke with almost 30 people and had all 30 tell me that
they couldn't afford me.
But because I didn't let those failures get to me and I didn't give up, I got a
yes at person #31, and I was back in business.
I'm making a bit light of it now, but at the time, it was the most painful thing in
the world to face all of those failures and rejections.
I'm sharing this though because I want you to see that for every success, there are many
failures that had to first happen to be able to get to the success.
So, shift your thinking from thinking about a failure as confirmation that you can't
succeed or that if you fail, that's it, game over.
Instead, think about it simply as part of the process.
Secret #2 Ask yourself - what's the worst thing that could happen?
Whenever I feel fear creeping up, this is the question I always go back to.
Really, what's the worst thing that can happen?
When I first started posting content on social media and sharing my knowledge with the world,
I was so scared.
I'm a huge introvert and super sensitive, so nothing about my personality helped.
But, I thought about it.
What's the worst thing that can happen.
If I write something and no one responds, I'll still be OK.
I'll have my job at the time, a roof over my head, and maybe people will laugh at me
and think I'm dumb, but if that's the worst case, that's not so bad.
I did the same thing when I thought failing in my business.
What if no one wanted to buy from me?
Ever?
Well, I remember thinking very clearly to myself even though at the time I really wanted
to leave my 9-5…
You know what?
Even if I can't make this work, I have a job.
Or I can get another one.
Or…
OK getting really honest…
I did think to myself.
If things get really bad, at least my parents will always have a place for me.
I never told them that, and I definitely wasn't hoping that would happen.
But I knew that ultimately, even if I failed in the worst way I could imagine, I'd still
have my life, health, and the people I cared about.
When you think about it that way, that fear is not so scary anymore, right?
Secret #3 - Think about your failures scientifically I remember reading a story about the billionaire
founder of Spanx, Sara Blakely.
When she was growing up, her dad would ask her and her siblings at dinner every night…
What did you fail at today?
And she credited that question with helping her develop an attitude of embracing failure
and seeing it as a learning opportunity.
That story really stuck with me, and that attitude has made all the difference in my
own business.
In fact, in my Employee to Entrepreneur course, it's an attitude that I try to instill in
every single student.
And when something doesn't go as well as hoped, I have epic spreadsheets to track everything
and scientifically figure out what went wrong so that it can be fixed.
I've seen embracing this final "secret" as one of the key differentiators of my most
successful students who are able to build the business and life of their dreams.
And I'm sharing this with you in the hope that you'll be able to do the same for yourself.
And those are my top 3 secrets to overcoming your fear of failure so that you can build
the business and life you want.
Because ultimately, when you weigh your dreams against your fears, I know that your dreams
are so much bigger and more powerful than your fears.
And that's why I also have another video for you, on 3 of the most toxic limiting beliefs
that will hold you back from achieving those dreams.
After helping over 1,000 students build their online businesses, I've seen these same
beliefs in almost every student, even after overcoming their fear of failure.
I've got your back and am not going to let that happen to you, so make sure you check
it out in the description below.
And if you want a step-by-step system for exactly what to do to build your own online
business, sign up for my latest masterclass on the EXACT system I used to build my first
online coaching business from scratch to 6-figures BEFORE I left my 9-5 job.
I'll show you how I built my first online coaching business using just my job skills,
from scratch, with no audience or anything...to $106K in 4 months while juggling my job.
I have it linked in the description, so go ahead and click there and you can sign up
for the training right now.
If you liked this video, please let me know by liking it below, and let me know in the
comments what your biggest takeaway was.
Don't forget to subscribe for my weekly videos, share this with your entrepreneurial
friends, and I'll see you next time.
-------------------------------------------
Desfazendo as Mágoas - Duration: 12:49.
-------------------------------------------
Sex Offender Dressed as Santa Arrested - Duration: 1:53.
-------------------------------------------
Air Force EOD Technician—Life as an EOD Tech - Duration: 2:57.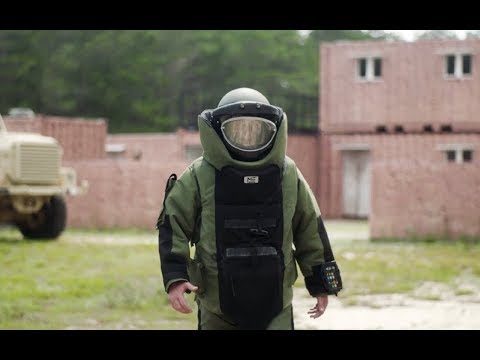
It's every little boy's dream to blow stuff up for a living,
and that's what I got to do. When I joined deployments were what was going
on, that was reality, things were very intensive, as far as people being gone
constantly, and that's what I was looking for, but with that career shift, with the
things slowing down, I think, refocusing on everything else EOD
has to offer is more than made up for it.
We have many different bases. We have more bases than anyone else. We also help
civilian bomb squads. We help them out if they have anything. We really get to go
into the communities more than the other services, so I've been able to go into
the mountains of Yellowstone and small towns where people find, you know, their
grandfather has a grenade from World War II. So we get to touch all kinds of
munitions that other services don't necessarily get to handle. When it comes
to EOD training it should be the bulk of what we're doing, so whether you break
that down by a day, a month, a week, whatever the majority of the time should
be training, and if it's not, well then hopefully you're doing things that are
operational. You're responding to incidents, you're doing range clearances,
you're out on the range blowing up things, because that's what we're
there to do on an Air Force Base.
What I would tell someone that was trying to explain to their family EOD,
is that EOD is about safety. It isn't about the danger. There is inherent
danger, but you need to trust that we will give you all the tools possible to
be the safest that you can be and you will always be focused on safety and
coming home. I take my role here as an instructor very seriously because I need
to impart some wisdom some motivation, you know, my experiences to these Airmen,
to make sure that the next generation is just as capable as the current. Most of
the guys that have re-enlisted, or are going to re-enlist, do so because they love the
work and the challenge and they love that teamwork. And that's hard to find in
the private sector anywhere. When you earn an EOD badge and you are now an EOD
technician you are a part of a family and we can literally go anywhere to any
service, and if you show up on a base and you find where that EOD shop is, where
that EOD company is, and you go in, and you let them know that you're an EOD tech,
they're gonna take care of you, whatever you need. It's just, it's a big family.
Anyone wearing that badge is your sister or brother, so I can go anywhere in the
country and that badge can get me- I have connections wherever I need to go. The
camaraderie is really important. We know that at the end of the day we have each
other's backs.
-------------------------------------------
Hwy 1 in Big Sur closes Wednesday as rain continues - Duration: 2:47.
-------------------------------------------
How to make money online as a teenager at home! - Duration: 8:33.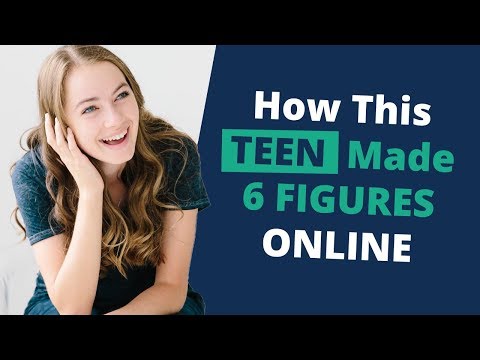
- Hey I'm sitting here with my daughter Makayla
and we're gonna do a little bit of a different
video this time around.
And how it's gonna go is I'm actually going to be
interviewing her about how she was able to sell over
100 thousand dollars as a thirteen-year-old.
That was the first six figures that she made.
And so I've got my list of questions right here
so that we can pick her brain to find out
how she made this a reality.
Are you ready Makayla?
- So ready.
(upbeat music)
♪ The best day of our lives ♪
♪ It's gonna be ♪
♪ It's gonna be ♪
♪ It's gonna be ♪
♪ The best day of our lives ♪
♪ It's gonna be the best day of our lives ♪
- You guys like our matching shirts?
Like we totally planned it.
(laughter) - We really did.
- Alright so Makayla first off tell us about your store.
Your online store.
What's it called?
Give us a little background of that.
- So my store is called iScarf2 with like the number two.
And we sell scarves online.
I started this when I was 13 years old.
We sold over 100 thousand dollars
in our first nine months.
It was pretty crazy.
I didn't quite understand how much money that
was at first until we broke it down into babysitting hours
and it finally made sense to me.
- Okay, now you mentioned "we".
So will you explain to everybody what the "we" is?
- So my sister actually helped with this.
She is not here with us right now.
- She's at Lagoon with her friends.
(laughs) - Yep.
- So Makayla what were kinda your roles between--
what did you focus on and what did Bridges focus on?
- So I did more of like the actual marketing kind of thing.
I reached out to influencers,
I talked to the manufacturers,
I did all the marketing stuff and getting the products.
And she took the pictures,
she named them and did everything like that.
- Yeah so Makayla was more front end,
Bridges was more of the back end.
- Yep.
- And it's kinda their personalities too.
Makayla is very outgoing.
Not afraid to be on a stage.
and Bridges-- she's a little bit more reserved in public.
Like she doesn't wanna get on a stage.
With her friends she's crazy loud.
(laughs) - Yes.
- So it kinda just fit their personalities pretty well
and they just fell into their own little groove.
Okay so tell me,
what is your favorite thing about selling online?
- My boss.
(laughs)
I'm the boss so she's pretty fantastic.
(laughter)
- She's got a lot of humility here.
- Yes I do.
(laughter)
- Okay what's the worst thing about selling online?
- So when we first started we actually didn't get
paid at first because we weren't selling anything.
So that was hard not getting money right away
for all the hard work we were putting in.
But then once we got it started and everything
was working smoothly the money did come in
and we got paid lots.
- Lots.
(laughter)
What do your friends say about this whole thing
that just happened or that has been happening for years?
- Most of them don't actually understand what it is.
Like they understand that I sell products.
They understand that I sell scarves online.
But they don't really know like the whole thing
that went into it.
A few of them do cause I have a few friends
who are also entrepreneurs.
But yeah.
- So if you had to do it again Makayla,
what would you do differently?
- I'd probably pick a product that was
trending throughout the whole year.
'Cause scarves are like big selling in the winter,
but not so much in the summer.
So like right now we are trying to sell them
but it's kinda hard to push a scarf in the summer
when it's too hot to wear a scarf.
And you wear--
So yeah.
Just pick something that's more fall,
spring, summer, and winter friendly.
- That makes sense. Okay.
And how do you balance this business with
school and friends and church activities
and just being a teenager?
- So I usually work on the weekends or like
a half an hour here or there.
I did have to take a year off for band.
My high school had the opportunity
to go to the Macy's parade last year.
So I had to put my business on hold while I put
all of my time, energy, effort, tears, blood, sweat,
everything into band. (laughter)
It was so much fun though.
I enjoyed it.
- That's another thing.
Just side note as a parent.
With her doing this business
we still needed to let her be a kid.
And of course I'm an entrepreneur and I'm like
no just keep going with the business.
But it was really good for me to step back,
let her do her band thing cause she learned quite a bit.
And now I mean she's super excited
about the business world again.
Right? - Yep.
(laughter)
Yep!
- Oh, how did you ship it all?
- My sister and I.
We did a lot of it ourselves.
But like during Black Friday and all the busy times,
fourth quarter all that,
we invited some friends over.
I did have to fire one or two of my friends
cause they weren't moving fast enough.
They weren't,
I guess, time efficient.
But most of them were good.
I enjoyed working with them.
(laughs)
- How did you know what to sell?
How did you know that you wanted to sell scarves?
- So it was kind of like the trending thing at the time.
I personally am usually very cold except for right now.
But I am usually cold and so I figured the scarf
would be a perfect thing to keep me warm,
keep other people warm,
but it was also trendy in the winter time
that would work and it would sell lots.
- Good.
And it did sell lots.
- And it did sell lots.
(laughter)
- How many scarves did you sell?
- Over 21 thousand scarves.
- That's amazing.
That makes me so happy.
(laughs)
Her business is in the homes of--
I mean people ordered multiples right.
But even if we chop it to a third.
Ten,
no seven thousand homes.
That's pretty cool.
Where did you get your money to start
Makayla, at thirteen years old?
(laughter)
- I did have to take out a wonderful small loan
from my dear mother
(laughter)
and father.
- How much money did you have in your
bank account when you started?
- Like a quarter.
(laughter)
- She was rolling in the dough.
- I was making bank.
- Do you remember how much the loan was for?
- A couple hundred dollars.
- Yeah.
- But if I were to do it again I would
not take a loan because they made me pay interest.
(laughter)
I would go work somewhere else and make the money
like babysitting or mowing lawns or
shoveling snow or something and make that money there.
(laughter)
And then do it.
- Are you still selling scarves
or what are you currently selling right now?
- I am still selling scarves.
They are still up and going.
I am actually working on a course to help
young teens, tweens, younger children
how to start selling products online.
Making it an easy, simple process for them.
I put some other products online
and we sold 26 of them.
So I made
a little over 500 dollars this morning.
- That's pretty cool
(laughs) - Yeah.
- We hope you like this interview.
Makayla and I have had fun.
This summer she's been home
and been coming down to the office
or just hanging out with me so it's been really fun.
I'm excited to see what she does in the future.
And you'll see her more on Facebook lives
sharing her experience with you.
And telling her story on how she made this her reality.
So if you're interested in building your e-commerce
business just like Makayla has done,
there's links up above or links down below.
Whatever social platform you are on
to find out the three secrets
that helped her make that a reality.
I've taught it to my sister.
I've taught it to my friends.
And I've taught it to thousands of women
and a handful of men
to make this process happen.
And it has become their reality.
So sign up, sign up.
- Sign up.
- What do they need to do Makayla?
- Sign up.
- Sign up. (laughter)
Alright we'll see you guys later.
- Bye. - Bye.
♪ The best day of our lives ♪
♪ It's gonna be ♪
♪ It's gonna be ♪
-------------------------------------------
AS VIÚVAS (Widows) | crítica - Duration: 8:19.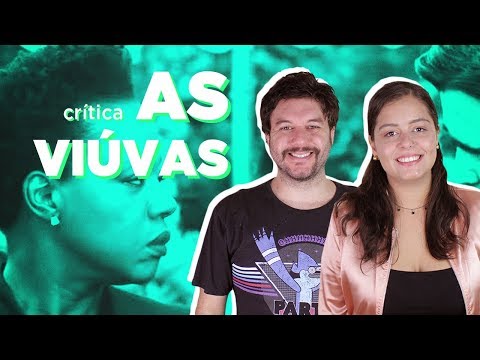
-------------------------------------------
Virginia family of Muslim teen raped, slain gets to question killer as part of plea deal - Duration: 2:50.
-------------------------------------------
Body found Tuesday in Lumberton identified as Hania Aguilar - Duration: 1:36.
-------------------------------------------
4 sprout food as good as gold - Duration: 4:41.
4 sprouted foods, are like ten golden .. Many people often say that sprouted vegetables are broken. However
The following 4 types of food are exceptions, it is also like ten gold .. First. Soybean sprouts. According to a study by the Institute of Nutrition
and Food Science, University of Agriculture, soybeans are highly nutritious foods, but they contain a number of nutrients.
Obstructing the absorption of the body. However, after germination, most of these substances will be resolved.
At the same time the content of nutrients is increasing significantly. Soybean seeds grow as fresh, the more smooth the more easily digested.
Especially suitable for people with poor digestion. . Soy sprouts, make soy milk
Or fried with mushrooms, are both the culinary options, both nutritious and delicious .. It should be noted that
Soybeans sprout for a shorter period, less than half the price of ti is best to eat. Second, pea sprouts
Among the bean sprouts, peas seeds are highly valued, in terms of nutritional value and utility to health.
Bean sprouts contain high levels of antioxidants. Meanwhile, the fruits, vegetables that we usually eat, only the average amount of vegetables
Bean sprouts have extremely high nutritional value. . Not only that, this kind of germ is also easy to process. We can use peas to make vegetables
Fried eggs or fried eggs are also very delicious .. Tuesday, sprouts incense thung .. Sprout shoots, possessing high content, valuable nutrients such as
Ca leo, because we have B2, with K.... The sprouts of cedar are quite crunchy and soft, we just use salt and sesame oil mixed with
There will be dishes with very good taste .. Wednesday, garlic sprouting .. Many people often question, Can germination germ can be used again or not? Actually
Only garlic sprouts are not moldy, do not change color is can continue to use .. Garlic shoots contain antioxidant content
Much higher than normal garlic. The content of these substances, the highest increase on the fifth day since the sprouting
Garlic has a sprout than normal garlic, the more effective anti-cancer, and slow the aging process. Besides, people are not used to eating garlic.
may try to eat the garlic sprouts. Germs of this type are especially rich in fiber, because of C, with A, C,.... Note, vegetables should not eat when sprouted
Potato: Potato sprouts contain hybrids. A bitter and poisonous substance. This poison will concentrate on the sprout, making the potato bitter
and toxic to the point of no use .. Sweet potato: Toxins in sweet potato sprouts, can cause vomiting, abdominal pain. If you see potato sprouts
Remove the germ, and soak in the salt water before use. Peanut: The process of sprouting, not only cause the nutrition of the peanut is reduced.
It also increases the amount of water that is easily poisoned, even increasing the risk of liver cancer. Ginger: When the sample or growth sprouting.
Although ginger is still spicy, it is dangerous because of the sulfur, which is produced during processing. Toxins in ginger sprout or crush
Particularly harmful to the liver, even the liver cells are poisoned, denatured, damage to the function of the secretion of the liver.
Some types of legumes: Although legumes are known to be rich in nutrients, they promote the development process.
And anti-aging, but also unavoidable exceptions. Some types of beans such as bean, bean, bean, bird's egg
Large amounts of zinc are produced when eating more harmful to the body. So, we should not eat the seeds of these beans
Please share, when you find useful content, wish you more health
-------------------------------------------
Salmos | SALMO 77 - "As Grandes Obras e Misericórdia de Deus" - Duration: 3:00.
-------------------------------------------
Popular beachfront camping spot is evacuated as a precaution - Duration: 1:36.
-------------------------------------------
Kate Middleton teria ido as lagrimas após implicância de Meghan Markle com sua filha, diz site - Duration: 4:30.
-------------------------------------------
Arsenal stars board plane to Kiev as it is confirmed fans CAN attend - Duration: 2:59.
Arsenal have gone through the painstaking process of contacting each and every supporter who travelled to Ukraine, with fans officially now allowed to attend the game
There was uncertainty over whether those who purchased tickets for the Europa League match in Poltava would be able to gain access in Kiev, given UEFA announced the stadium switch at such short notice
Yet following talks with European football's governing body, who moved the match because of 'security concerns', Arsenal have confirmed their near-500 fans will be able to get in to support their team
Supporters of their opponents are furious about the switch as martial law, which was imposed by the Ukrainian parliament on Monday, is not active in the city of Poltava
Some of Vorskla Poltava's fans have even called on their club to snub the European tie and refuse to play, despite the potential repercussions
Vorskla are still planning to take part but thousands of their fans are unlikely to travel, some in protest and others because they are unable to make a 420-mile round trip in the adverse weather conditions
Anyone who attends will also have to book accommodation in the Ukrainian capital
As well as several supporters, some Arsenal staff, such as security officials, were understood to already be in Poltava
Speaking on Tuesday, Vorskla head coach Vasyl Sachko said: 'I am not the organiser of the tournament
The club does everything possible to play at home. For us, let it be an additional motivation
'We need support from our city and our country. We protect our honour and this situation is an additional motivation
'Ukraine's president called for martial law to be imposed in the country because of rising tensions with Russia, whose coastguards are accused of seizing three Ukrainian ships in Crimea on Sunday
Arsenal are already through to the last-32 of the Europa League and can top their group by beating Vorskla
They defeated the Ukrainian side 4-2 at the Emirates Stadium in September.
-------------------------------------------
Policy Optimization as Predictable Online Learning Problems: Imitation Learning and Beyond - Duration: 1:10:13.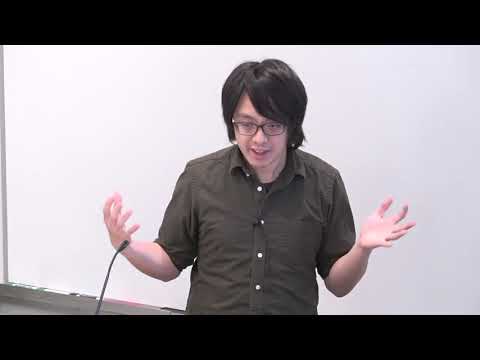
>> It is my pleasure to have Ching-An Cheng here,
visiting us from Georgia Tech today.
He is PhD student finishing in about a year.
He's doing a super cool research
at the intersection of machine learning,
control to imitation learning,
reinforcement learning, and so on.
Specifically, it is a very cool combination of theory,
again test paper in AI starts this year and practice,
where he's applying his approach on actual robots,
rovers and he got the best systems,
he was the best systems finalist
that are assessed this year, the robotics conference.
Anyway, so without further ado
I'm going to handed over to Ching-An.
>> Hi, good morning.
So, thanks for having me here.
So, today I want to talk about
my recent research in policy optimization,
and specifically on the idea of
treating policy optimization as
predictable online learning problems.
So, these direction on research is
motivated by our research imitation learning,
but we recently go
beyond that and trying to further extend that to
also to reinforce learning also
beyond other optimization problems.
So, if you go into details,
let's talk a little bit about myself.
So, I'm currently a fourth year PhD robotic student
in Georgia Tech, advised by Byron Boots.
So, my general research interests lies
in the intersection between machine learning,
optimization control theories,
and it goes trying to build more efficient robots algorithm
with theoretical foundations.
All right. So, my current research are in three parts.
The first one concerns RL and imitation learning,
about just trying to build up everything to learn policies.
The second part about is about trying to
use very large-scale Gaussian process to model uncertainties,
and let say with the linear complexity,
and the last part is about the control and planning.
So, I want to design everything that can combine
the best part of planning and control
and their properties together in a single framework.
So, and today I'm going to talk about,
an online learning approach to pause optimization.
So, in online learning,
as we probably know,
is a framework to analyze online decision-making,
the way you work this there is a repetitive process.
So, each iteration and let's say the learner makes
a decision Pi n alpha decision says capital Pi,
and in the policy optimization context,
Pi n will be the policy that the learner will currently have,
and and capital Pi will be the class of policies.
Now, once they learn the step policy,
you will run that in the environment,
and the environment will give returns a cost function,
loss function L_n, and that can
depend on the Pi n which the learner chooses.
So, once the learner is determining and
then the learner would suffer a loss function and the end,
this will repeat for minimum iterations.
In the end we care about bounding the performance
in terms of accumulating laws that the learner suffers,
the solve L n and Pi n.
Then, because in general,
this L_n can be adversarial,
so probably we can only bound the regret,
in terms of these community loss.
So, we are just comparing that,
versus say the best decision that you
can have chosen in hindsight.
So, what do I mean by policy optimization as online learning?
So, on the left-hand side, we have
the policy optimization problem,
like training a policy to do something,
some job that you want to solve,
and on the right-hand side we have
the theoretical framework for online learning.
So, in here, policy optimization has an objective function.
So, in order to convert that to online learning,
we need to define what we want to
have as a loss function in our learning,
and once we have that loss function,
we might know some property about those functions,
and they are going to design some online learning algorithms.
We're going to use that,
and then we're going to use the technique that we know of
online learning to analyze the algorithms
and then at the end we get some performance bonds
about the policy optimization
process that we care about at the end.
So, it's like converting policy optimization
to online learning by some proper conversions,
and then solve it there and then bring
the performance back to the original problem.
So, the thing I will talk about today is that the first one,
I will review the classical reduction that we
have from imitation learning
into adversarial online learning problems,
by seven rows and L and so,
the second part is that,
I would actually argue imitation learning is not adversarial,
so the previous reduction actually
low sign information and it's not optimal.
Then, this leads to the idea of using
prediction to make it better,
so to piggyback in terms of analysis
and also in terms of designing better algorithms.
The last part is that I'm going to present
a reduction frame work, it's called PicCoLO.
So, it's a general reduction framework to
convert predictable analytic problems into adversarial ones,
and so you can use standard technique for
adversarial learning to solve that ultimately,
and that can be applied for imitation learning
IL even beyond those scenarios.
So, let's go to post optimization.
So, post optimization here we
care about something or reinforcement learning problems.
So, we want to find a policy Pi,
that has good performance in terms of [inaudible] function J of Pi.
Here that J of Pi for example,
can be defined as the average of the expected accumulated loss,
and where the expectation is taken on
this trajectory distribution generated
by rounding the current policy Pi.
So, these are the final IL problems,
but you can extend the same techniques
to infinite horizon one as well.
So, the way we're going to after this problem is done,
we are going to exchange the order
between summation and expectation.
So now, this we can define
a more actual generalized version
of an average state distribution.
So, basically what it says is that
the loss function that I want to minimize,
is the expectation of the instantaneous codes
over this data and action distribution generated by the policy.
So, here have two proponent parts,
so policy will generate a state that it raises and it was a policy
will generate the action that
it wants to take as a particular states,
then it converted that one to minimize.
So, why do we want to consider imitation learning?
So, the reason it minimizes
because RL is just too hard to solve in general,
and also because RL is not a problem I want to solve,
let's say for robotics.
For robots, let's say we want a robot to achieve some task,
RL is purely a mathematical formulation
that we have about this process.
This is not like we are not stopping
that mathematical problem in our application.
This is just a way to find that problem.
So, if you're thinking like that,
we actually have more information about
the application on the problem that we actually care about.
We have this prior information.
So imitation learning is trying to use
this prior information to speed our policy learning
in the form of the expert demonstrations.
So, this aspect can come in many different forms.
A common expert can be like
human demonstrator which we know about
like the task I want to solve,
let's say you want the robot to grab a code,
you can demonstrate how to grab the code yourself.
But not all experts or algorithms or heuristics,
because we have been doing research in AI for many decades.
So let's say, we simplify it a little bit.
I will remove some constraints or we add more sensors.
Now, it might become a problem that we know how to solve.
Let's say we discretize the role,
and just make the space base even smaller,
we can actually do planning optimally.
So, this kind of heuristic
actually can be used as another form aspect,
in order to speed our policy learning
for the only problem that we care about.
So, the goal of imitation learning
is trying to minimize the performance difference
between the learners policy and the expert policy,
and by a performance difference lemma,
this can be written as a equality.
So, the difference will be equal to
the expectation of the advantage function,
with respect to the expert policy,
and over that stay
and action distribution generated by the learner.
So, because the finish function basically measures how
an action is better than
an Expert Policy on that particular state.
So, if you take the expectation over
the state action pair of given by the learner,
then basically it tells you that performance difference.
So, imitation learning will be
trying to minimize the thing on the right-hand side.
But usually, we don't have the definite function directly.
So, we might want to minimize its upper bound instead.
So, let's say we have another distance sensor like,
normal distance function between two policy Pi and Pi star.
So, we can upper bound this gap,
with a constant times the expectation
of that distance function over the state needed by the learner.
So, now we have a problem that we potentially can solve.
But what is this problem?
So, in this problem,
we first have to define this distance function,
and there are many ways to do that.
For example, you can use an estimation of
that definite function for example,
or you can use as the action different inside the square loss
between learning and the expert action.
Or you can use KL divergence
because we are minimizing statistical distances.
Another thing that people can also use is that,
you can use an adversarial trained neural network
to learn how the loss function,
so that it works in a more robust setting.
So, there are many ways to get that D,
and then you get a different constant C there.
But there is still an RL problem,
and the memories signed for that is because in here,
we are still considering the state distribution
as a function of our policy.
So, let's we want to take the gradient,
we have to consider the bifurcation through dynamics,
and that's a difficult part.
Although this problem might be easier than the origin one,
let's say original problem have a cost function C,
and that might be sparse
but we define it's D function which might be dense.
So, this is RL problem.
So, we define our upper bound,
we get the new RL problem,
but that might be easier,
but it might be still very hard let's say for robots
because you'll have to interact a lot with the environment.
So, another way to further simplify this is that
because we know the difficult part
comes out of that state distribution.
So, let's assume that's going to be fixed
regardless of how we change the policy.
So, what we end up having is that,
lets say we have some policy Pi n,
and we get some state distribution,
and assume that's fixed,
and we just optimize the part
that's independent of the state distribution.
Now, we have this loss function and that's very easy to optimize.
It's almost like statistical learning.
But there must be a cost in here,
we must have some drawbacks.
The price that we take is that
originally the IL formulation is
a global upper bound of the problem performance difference,
but now for this one, is only a point-wise upper bound
which only holds exactly at the Pi n policy,
which is the one you defined that's the distribution.
So, what it means is that
if you minimize this loss function directly,
it will not ensure you to minimize the performance difference,
because you only host at a particular point
not the entire policy class.
So, we can not use that as a super ascending cost function,
but we can actually use that as an RNN loss function,
because the thing on the right-hand side of the upper bound
is actually the loss function
that we want to bound in our learning.
Its exactly the sequence of loss function
that we have in our learning when we
designed that regret for example.
So, we can use as a learning problem, loss function.
So, now let's treat that as
a learning loss function and
the classical element that we can use,
that probably the first one you will know
in our learning is Follow-the-Leader.
So, if you apply this idea in
imitation learning about the loss function that I defined,
that will lead to the very classical element then it was
a very practical practice are
called data aggregation or value aggregation,
so a staggered aggravate wishing me my hurt.
So, what it does is just using
that loss function and just to Follow-the-Leader.
The way it works in practice that you have some policy,
you use that to run a policy in the environment,
you collect some samples,
you defined the loss function for this iteration,
and then you use that to sum all of those functions together,
then you update the policy by min and minus n of them.
You do this again and again and again and at
the end you have some positive sequence and that might be nice.
So, because of this is just as very
classical algorithms we have very simple analysis.
So, if we assume that IL in loss function is
strongly convex and Lipschitz which is nice,
but nothing that can have, then of course you can get
the standard analysis convergence in our learning.
So, you see that using
that inequality and combined with
a regression analysis in our learning,
we get performance spawn in
the policy sequence reassess that on average,
these performance sequence will
converge to the actual performance,
plus in a rate of let's say of
log n over n plus some tiny Epsilon.
That Epsilon captures how expressive this partial class is,
and this is the minimum that you can achieve
by minimizing all this sum of sequences.
So, basically sum tells you whether there is a
parsing your policy class that can imitate
the expert well in the almost globally.
So, for example, if you're a policy class
contains the extra policy, the Epsilon would be zero,
which means that eventually,
we'll converge close to the actual performance
after some iteration on average.
So, we can of course,
we'd have Follow-the-Leader but we also have
a punctual I first or they're always in online learning.
We can take gradient Mirror Descent or farther regress leader.
So, if you do that, go to some
other like everything in notationally like
AggreVaTed or DAggereD and of
course just as then any other learning techniques,
you get that similar performance bond.
So, if you visualize either the day,
so why you do it is that you have
a cat heathen trying to imitate a lion expert,
and the behavior you have here is on errors,
so you might lets say the blue curve
is the loss function of the IL problem.
You might get some oscillation in between,
but eventually you will converge
close on average to the excellent performance.
So, the benefit, let me summarize.
So, online learning approach to imitation learning allows
you to use optimal experts as a demonstrator,
so as to speed up personal learning.
We can show that especially when the expert policy is not trivial,
let's say it's just better than random policy that you will have,
let's say when you randomly initialize a neural net.
Then, you'll converge faster than an IL,
the main reason is because in here you
minimize the relative performance gap.
So, is invariant to the absolute scale of the original problem,
you're pressing cutting the performance gap
based on the difference,
in some fixed rates almost.
Another reason is because the gradient
you use in imitation learning,
is much less noisy and doesn't have much bias.
So, in here, you ignored the state distributing chance,
so don't have to do that very complicated part.
Also in here, you don't need to estimate the value function,
you have some p function which we might
know how to take derivative so it might be able
to take analytic gradients for example.
So, that's why it converge faster,
a lot faster actually.
But, so, everything seems very nice. Okay.
>> Do I need to have access to
the experts policy by starting some parametric form?
>> No. You only need to treat that as a black box function.
So, that it can just query
the demonstration for a particular state.
>> Even that is able to give us
low gradients, low viral gradients?
>> Yeah. Because the memory size because in IL we
need to estimate that value function or the advantage function,
and that's half the future,
because in here we are ignoring the
derivative through the state bar.
So, you don't have that accumulated part,
you only care about instantaneous one.
So, that's the main reason why he
has like very nice gradients compared with IL.
So, feel free to interrupt me if you have questions.
>> Does your signal platform in this setting
where you only have finite number of
expert demonstrations notch really applied function
in caret shortly going to infinite many times.
>> Yeah. So, it's doing nice in the sense
in that sentence sometime they say you have human demonstrator,
let's say, is not very convenient for you
to just query ask questions.
>> Yeah.
>> You can do the
deciders more centralized behavior occur only one,
and you get similar properties but
theoretically is worth and is unlike interactive version,
but it might still be better than the IL part.
It's at the beginning of learning,
because at the end you will see it like there is
optimality of the expert
because you cannot perform better than that,
and also converts it into later on will be slow.
But, at least at the beginning of
the comparative performance or you
get a number of examples that you
have in session learning versus the number of
that experience that you have to interact environmental IL,
that part the in session one will be faster.
But, the question is that now,
"This is the hour that we have been using for years,
it is 10 years at least and what can we do better than that."
So, in order to think about this problem,
let's revisit the reduction. Sorry.
>> Can I ask some other question?
>> Yes.
>> In your framework,
can you do better than the experts?
>> So, if you want to do, we can discuss it maybe later,
I've taught Picasa, so then that become
an ideas I wanted to do better than an expert.
You need to have some signal
from the loss function and care about an IL.
If you somehow mixed the gradients
or mixed at the function together,
or let's say do machine learning and spiritual IL,
in some sense like that, so you have
some signal from that and you can do better than the experts
and still much faster than IL alone.
We can talk about that flying if you want.
So, can we do better than what we can do right now?
Let's revisit what we have done
using that redundant idea, that's three steps that we have.
So, we define loss function,
algorithm, and performance bound.
So, in the current setting,
we had the loss function which is our performances in IL.
We'll reduce that into these online loss function
by ignoring this stay where it says the policies.
Now, we design algorithm,
use optimal algorithm in
the online learning literature, for that setting.
We also use the optimal regret bonds that we have,
up to a constant of course.
So, everything is optimal.
So, what can we improve?
The thing is that this only
optimal when the permanent is adversarial.
You're integrating these things on
an optimal when the loss function
is changing arbitrarily and in that sense.
But, what if the problem is not optimal?
Is the loss function that we have is actually optimal?
I mean, adversarial. Sorry.
So, here I'm going to argue why that's not adversarial?
So, the first thing is that if you look at this loss function,
the thing inside the expectation is the same for every iterations.
You are trying to learn from the expert,
and in most of the settings,
the expert is not going to re-add adversarial to you.
You might have that scenario, but probably that's
not the case you want to apply mutationally.
So, that part is not very bad.
You're either left with the same or it's trying to be nice to you.
All right, and also,
the other part is the state distribution.
So, in online learning,
in the previous reduction,
we have trained that adds the arbitrary component
that might appear in this like online loss function.
But that state distribution
actually come from the same MTP process.
We're not changing the robot,
the environment is not going to react
adversarily to our policy change.
That might be touring other cases in some game for example,
but not usually in the case we care about.
So, that power has some continuity that we can leverage.
Right, some ideas might,
I hope they convinced you that
this loss function is not completely adversarial,
but actually, can be predicted using past information.
For example, interacting environmental asses,
you see some state transition pairs.
You have some knowledge about dynamics,
which less how you can predict the loss function.
For example, you can even query
the aspiring lets say in simulations.
Then, the thing inside the expectation is actually known to you.
So, that part is not adversarial.
So, this is actually somehow an online learning problem,
but it's a predictable online learning problems
where the loss function can
predict from the past in some form and it's partially.
>> For the sake of being adversarial,
but they're basically they trying to teach you by leading
you to parts of
the state space where do you think you know doing well.
>> Yes.
>> Is that considered adversarial at some sense?
>> So, I think we don't have much theory about,
so currently, most of the learning,
assuming that they ask for,
I have a fixed distribution,
you can have many learning expert,
but that distributing is fixed,
and that doesn't change with the learner, right.
The part that become the learner.
But it's interesting to accurately think
this resonance a single player online learning problem,
but also as maybe collaborative game.
For example, you have asked for and trying
to do well together so the learner can learn well.
It's interesting too in that scenario,
I think there are some few papers about that.
That's also why I advocate,
but when you can get beside the expert yourself.
But that scenario becomes awkward,
in the sense that when you are lets say,
you ask for is a human demonstrator.
The thing is trying to help you but it's actually hurting you,
and that's where the theory becomes vague.
Yes, but in here I will assume that it's
expert is just fixed for now.
Yes. So, now we have this as our previous diagram,
that if now things become predictable,
the algorithm then we had,
the bumps that we have are no longer optimal.
So, now this become question most.
How do we design everything for the setting?
How do we do analysis?
Okay. So, for the rest
of the talk, I'm going talk about two parts.
So, the first part is trying to have a better analysis for
the previous algorithm is
a Follow-the-Leader in Dagger or Aggravated,
by using the fact that
this loss function is predictable in some sense.
The second part is, to design better algorithm
that use that particular information to make learning faster.
Okay. So, let me talk about the first part.
So, this is my S that's packed with this year,
so the main motivation is that,
there's a gap between theory and what we do in practice actually.
So, in theory, online learning the analysis,
it tells you that, we have our average convergence.
Right. It doesn't really mean that less than is the best one.
So, what it means is that,
you can either run then you stop
the sequence and then may angst petition to
give them the one that you want
or you need to keep all the sequence,
and at the end identify the best one among them
and they will have the thing you can have guarantee on, right.
But as a practitioner and probably the thing you will implement,
it just run Dagger from the number iteration and you'll stop,
and that number iterations is not chosen randomly,
just something you've think that's like,
you pivot out during the week,
that is a number of external that you can do and
that's your budget, and just run it and stop.
Then you use the last policy as
the one that you think that's the best one.
The intuition is because,
I think in the last one is trying more data.
So, potential should be the best one, right.
But is this really true even in practice,
there's a policy actually improved
monotonically with let's say we're going to do
more iterations of Dagger or Aggravate.
If you look into different papers, you find different answers.
So, if looking to process paper
like the one we proposed that you find yes,
become better naturally during our experiments as well.
But there are other papers which can construct artificial,
actually [inaudible] cases where this is not true,
that the error can increase when you do more interregional Dagger.
Most of the time this is because there's
a gap between your policy class and the experts policy.
So, if that's true, then this is more likely to happen,
but if that's the case then usually you
might see the other one, but it depends.
>> [inaudible].
>> Yes, and then the many results
that we have in this paper is that,
actually we can show the convergence of the last policy
of this idea theoretically,
and we have a condition and a bound for that.
Right. So, this is only
visible when we know the problem is predictable
because in general ever in online learning there's no way
for you to show the last convergence for any any sequence.
So, here we're going to use the fact that we're going to
redefining the loss function like this.
So, the way we're going to characterize this structure,
is that we're going to define a bivariate function,
a function with two arguments.
So, the left argument of
the AF-Dev function is going to be
the policy that determines [inaudible] distribution.
On the right will be the one that
you put into that distance function.
Right. So, if you can compare
this definition with the definition
of the loss function that we have,
you will see that LN is just
actually just putting Pi n on the left argument,
and if the gradient that you will see,
when do learning will decide online learning parts.
We will be just taking the partial derivative of capital F, right.
So, now we have
a maybe a better way or
more close way to describe the loss function that we have.
Now, we are going to make some assumptions,
reasonable assumption and that can give us
more information about what actually involving the [inaudible] process.
So, the assumptions that we're going to impose is on,
how fast the gradient that we
see from online learning is going to change,
when we change the policy desk used to collect the data.
So, we see that the partial
derivative corresponds to the gradient
that you were seeing online learning,
and now this encoding basically says that "Is going to be
continuous in the policy that you
use to collect data" with some Lipschitz constant beta.
We are going to talk about what that is theta.
Alright. So, what we show,
is that there is a single stability constant which
we call theta which is the retrofitting beta and alpha,
and alpha is the strong convexity of the online loss function.
So, you can think that the convexity will provide
stability and but it's betas large,
the gradient change a lot when
you just perturb the policy a little bit,
which means that the parent is very sensitive,
then it become unstable.
So, we show that with less than one,
you've wrong Dagger Aggravate, you always comes first,
the less policy would be the best one,
but if beta is larger than one,
there are some cases where it will always diverge. Alright.
So, the first result that we have, yes?
>> Just a clarification, this has nothing to do with
the complexity of the policy class
because it's more to do with the dynamics.
>> Exactly. Yes. Yes.
>> Okay.
>> Yes. So the first result that we
have is a convergence
of upper bound outputs performance of the last policy.
This bound is actually non-asymptotic,
let's simplify that in these slides.
Then so, what happens here,
we show the last policy is bounded by the Epsilon performance,
plus N Epsilon, plus N to the two times theta minus one.
So if you see that,
if theta is less than one,
then the thing on the blue balls will converge to zero at the end.
That implies a conversion with it at less than one.
Here, the delta Epsilon is a little bit
different from the previous Epsilon as I'll explain.
So here, delta Epsilon is
the minimum value that you can achieve for a single loss function.
If you compare with the previous one that you have,
the previous one is about an average convergence.
Previous Epsilon is about the minimum that you can
achieve among a set of loss functions on average.
So you can imagine that if your policy
can only imitate the Epsilon locally,
then the new delta Epsilon will still be small,
but the first Epsilon that you have
in there might be very large because there
might not be a good policy that can
mimic the expert globally on all states that you visits.
So here, you have a bound
that's interesting in the sense that it is dependent,
is tighter in the sense that it's half of
Epsilon that describes the thing
that you might care about more in practice.
On the other hand, you have the previous first Epsilon,
which is a little bit too conservative.
Also, we have a convergence rate that's
dependent on the difficulty of the problem.
So we can actually show there's a lower bound,
which matches around the upper bound.
So that's why this convergence in terms of [inaudible] theta is tight.
We can now improve that
unless we have full information about that problem.
This is even true when the policy class,
contains a global optimal policy
for the earlier problem in the convergence problem.
So this is a very strong result in some sense.
But this is set because we are showing
that we have some problem and
its dynamics determines that theta, right?
We don't have any control of what theta is.
Given that dynamics, we have
some problem where you are
saying that wrong integrity will always divert,
for example, and some of it is nice.
We don't have any control or guarantee
about whether we want to use a less policy,
or tried to kill all of them, and this counts that.
But it turns out that we can actually
stabilize a problem by adding regularization.
So if we think upon it a little bit,
by adding regularization to the loss function,
then for this new problem,
we can have an effective new delta theta and
that's going to be less than one if you add enough regularization,
which means that if you solve the new problem,
then it will always converge and at the end,
you can just pick the last policy without really doing the stuff.
>> So just to make this completely clear.
So even if you have the policy class that
learners using contains the policy of the experts,
the data can diverge.
>> Yes, in some real case.
>> But then, so the proof Ross from 2012 theta,
there seem to be contradiction?
How then this leads to contradiction?
>> Oh, there's no contradiction. So the thing
come highest into that f Epsilon.
So in that case,
the Epsilon will be very large for the original formulation.
That can divert and Epsilon will go to infinite.
>> Okay.
>> Also, here is a little bit different.
So here is like Pi
contains a globally optimal policy for the insertion process,
but that might not be the expert policy.
So the way we construct this context sample is that
a policy class of the expert is different from this policy class.
>> Okay, okay.
>> But there's still a policy in this policy class
such that it gives you zero in potential learning error.
>> I see.
>> Yeah. So we don't know whether this is true when
this policy class contain
exactly the expert policy. I think that's not true.
Assuming that's the case,
this Epsilon can be zero for that scenario.
But, in general, you don't know whether that's true or not.
So let's say, there's a difference between let's say,
the experts just not strictly inside
a policy class and for that case,
we can construct a problem, where you will divert.
But still, this policy class that we have actually have
a global policy and it can be actually solved in linear time,
which means that they convert exponentially
fast, it just take gradient.
Do gradient descent with ordinal aisle problem.
I mean, the RPI formulate with upper bound.
So does it answer your question?
>> Yeah.
>> So theta is heading to the Epsilon.
So I'm going to talk about
two approaches to stabilize the problem.
So the first one is actually a way to justify
the heuristic that Stephen Ross already proposed in their [inaudible] paper.
So in honoring the problem that we talked about,
we are saying that the loss function for
each iteration is defined using the current policy.
But in fact, that's not usually the case that we do.
What we do in practice style when we collect the data,
we're going to run a mixture of
the current policy and the expert policy.
In the original proof of their paper,
they don't provide a constructive reason.
They just say that when you do this,
it will not hurt the regression.
So it doesn't tell you why you should do that.
Here is an expert explanation of why that makes sense.
So what we show is that
if you mix a policy together, and let's say,
for each ties that you have some probability to
use the expert policy instead of
the current policy to collect the data,
and let's say we call that a mixing rate.
So we are saying that if the mixing rate is high enough,
which means that you are going to start
with high enough probability,
we are going to use the expert policy to collect data,
and the new problem will have
a delta Epsilon that's going to be less than one.
Because you can imagine that when let's say,
the missing row is just one,
always use the extra policy,
then you have a supervised learning problem,
and it's always stable.
But you have a cost.
You'll have an additive constant cost there,
and that one is going to be proportional to
the mixing rate that we're going to use the expert policy.
So there'll be maximized.
Let's say, if you always use the expert policy.
So the higher the probability you put on
the expert policy because you're going to see
a different distribution later when you come to visit,
then that constant bias would be larger.
But if you probably choose that,
as long as that's larger than some threshold,
then the probability will be stable
and the constant might be a little bit smaller.
So that's one way to stabilize a problem and it
justified the heuristic that people have been using.
So another way to stabilize problem is actually better.
So the way it works is just that previously, let's say,
we have the analog function for imitation learning,
and that's collected on the current policy.
Now, let's say, we consider a new online learning problem,
where the online loss will be
the origin loss function that we defined for the online learning,
plus the loss function collected under
the expert policy ties and waiting on that.
So the cost on the right hand side is the thing you would
do in the supervised learning approach for imitation learning.
You just add them together and times some weight.
This is actually being used in the literature,
especially by people from Berkeley.
So I have some empirical favorite bulbous,
you're just mixing the ingredient
together and apply that on robots.
So we showed that if the weight is larger than some threshold,
which is partly depend of course,
and we don't know how exact that is,
but it's larger than threshold,
then the new problem will have
a delta theta that is strictly less than one.
So it will always converge.
If you compare this result
with the previous one with randomized mixing,
then here, the cost that you pay becomes multiplicative constant.
So you will have a constant bias.
So that will magnify that delta Epsilon that we have,
which is already smaller than the original Epsilon.
Then of course, you can imagine that this constant will
become larger if you put more weight on the expert part.
So the way go to larger,
then this constant become larger,
and because the reason why it doesn't have
a constant bias is because in this formulation,
no matter how you highlight the weight is,
you'll always have some component from the current policy.
So you will have the cost advisor who has
an experience from current policy.
Then, everything shifts into the constant.
I mean, the multiplicative constant on that,
and what it means is that if you want your problem to be stable,
so you choose a large weight, for example.
But you can still have better performance than say,
just by increasing the expressiveness of the policy class,
and this partially explain why this technique work
with the training imitation learning with our deep neural nets.
So you add those gradient together, make the problem stable,
and now, you are safe to say that I can use a less policy,
and then only thing I need to worry about is trying to
use a policy class that's expressive enough,
so that constant factor doesn't matter that much.
So let me summarize what I did in analysis.
So we showed that the convergence of
value aggregation or disaggregation is
actually problem dependent if you don't turn the problem.
You might diverge or converge.
There is nothing for you to control.
But if you regularize a problem,
you can always measure that will converge,
and that creates some way to justify
the heuristic you'll have been used in practice theoretically.
Recently, like actually, in December,
there's another paper, which extend this idea
about trying to think about prediction,
about how fast the gradient would change in terms of policy,
and you can show similar convergence result for
the first order approach I talked about for imitation learning.
Because if you just take gradient [inaudible]
and you follow the leader.
It can still similar convergence.
Also, shows that the convergence also depends on the test data.
Okay. So that finishes the first two-thirds of the talk.
So then, the next thing I have mentioned
that now we show using prediction,
we can have tighter analysis which might also
explain the things that we had been doing for years.
So that's one thing, but this entrench our algorithm very much.
So the next thing is that can we use this prediction information,
particular information to design
even better algorithm that can converge faster theoretically?
So how do we do that?
So, for the rest of the talk,
I will switch back to focus on the average convergence for now.
So you might have a larger Epsilon,
but let's focus on that.
So, the thing we're trying to think about is that what do we
actually mean by using
predictable information inside an algorithm.
Let's say what kind of representation or how do we
actually represent particular information in our algorithm.
So to do that,
let's try to get some intuition about what kind of
formation that we actually need when we
are doing policy optimization.
So, let's consider a very idealistic scenario in irony.
Let's say in each iteration,
I not only know the current loss function,
there is someone else give me the next loss function.
So if I have that information,
I can actually update
the policy by an algorithm "Copy The Leader",
which is just adding the loss function of
the next one in
addition to the loss function sum
goes on I have been follow the leader.
So I always have one step ahead.
So in that case,
if the next [inaudible] is exactly the
same as I will experiencing it later on,
then we'll have zero regret.
So which means that convergence
on average will just converge in one step,
it's always zero and
that you will solve the problem completing one step.
So this is an ideal scenario,
which may not be doable.
So let's think about how do we actually
implement this in this setting.
So this is not doable at all in
the general adversarial case
because you don't know the next [inaudible] and then he can be arbitrary.
This always used to think about analysis.
But in this particular problem,
this might not be visible.
So to think about that and the reason for
that is because we define this loss function
using these bivariate function capital F and
other capital F is just use across all the iterations.
So, if we know that capital F,
maybe we have a way to know the next loss function.
Because that capital all the information that we need.
The way we do that is that we can look
into the optimality condition of these update.
So that is say can written as a virtual inequality,
which says that the direction of that gradient
of some of those loss function should be in the direction of,
should have a echo direction
for other territory in the policy sets.
So you want to find a policy Pi m plus one such
that this inequality is true for all the passing your class.
Does basket automatic conditional order of the Army.
Now to do that, using the condition,
it actually give us some information about how we can
use that capital F to be the leader.
So, if we know capital F,
we can use definition that the gradient
of the next loss function is equal to the gradient
of the partial derivative of capital F at Pi m plus one.
So now, if we putting that into this inequality.
Now this is actually a problem that we can solve because we know
all the variables that capital F
and the gradient of previous loss function here.
If we can solve this version of inequality,
then the solution that we get and when the capital F is exact,
then we will be exactly doing be the leader algorithm.
So, which we know
capital F and if we can solve this inequality permanently.
You can just think that as some sort of equilibrium point problem.
Then we'll be doing exactly be the leader and we will
suffer zero regrets and everything converted in one iteration.
That's very nice, but in practice we
don't know about capital F otherwise we have solved a problem.
So, let's think about approximation.
What do you want to approximate?
If you look at it here,
use the information of this partial derivative.
So let's say we have a mapping,
which such that given any policy Pi,
it can approximate that partial derivative.
So, let's just replace that and then we replace
that and then put that into
this virtual inequality and we solve this problem instead.
So as long as you can imagine that,
as long as that prediction is I count how close,
then you can still doing almost as well as be the leader.
In this paper, I can show that we
can actually learn their loss online and still be sufficient.
But I will not go into the details,
but the thing I want to tell you here is
that this algorithm which
I will call MoBIL model best imitation learning,
but here the model is about
a way to predict that partial derivative.
So, the model is not just dynamics model as you might thought.
Here, we're talking about model
instead of predictor on learning is a way of mapping
that you can predict this derivative that we care
about when we want to be the leader.
So, we're going to call this mapping predictive model.
It's a way to predict where it is and
that it's a better value mapping.
In other words that if we have a matching,
if that predict exactly and we can
solve that virtual encoded to be the leader,
then we have improved convergence.
But there are practical issues.
So, what we know is that this formulation suggest
that there are some information that we can
leverage to make algorithm better.
But that algorithm,
I remind you that you don't know how to solve this one.
This requires an iterative process
to actually solve for the solution for
the inequality and it's
not trivial and it might not converge as well.
So there's a practical problem,
one idea of contextual version of the thing we can do,
but probably not the one that we'll go into implementing robot.
So, we need to have a more practical version.
So why might you design come with
those algorithm is that we can look
into the literature of predictable online learning problems.
There are already some algorithm that's unfortunately
algorithm in like mirrored products,
optimistic real descend of how the reader
with further rigorous leader with prediction.
Maybe you can use those algorithms to design.
In previous target we use follow the leader.
Let's say, we use all these new algorithms for
that specific setting and design
new imitation and the algorithm for example. Is that a good idea?
But the problem about this approach,
I'll argue is that this problem is actually less
well studied compared with the adversarial literature
and they are usually more complicated.
They have a nasty update rule and usually requires
certain fine tuning of the step size for it to
work and those finding SSI actually requests bunch of constant,
which we don't know in practice.
So that makes for
application scientists want to implement that
those algorithms on a robot becomes very, very difficult.
So in practice, let's say we are
using function like modification of
those theoretical algorithms in practice and so we
need a definite algorithm that can work robusting practice.
Those algorithm should also be very easy to
tune and to understand as well.
This is more like an issue,
in general predictable on learning not just on imitation learning.
So here we are reducing that to particular learning and now we
realize there's an actually practical algorithm
that we can use in practice.
This might explain why people haven't been
thinking about converting property into
predictor on learning problems because we don't have
well study theoretical algorithm for us there.
Scientists understand that, but not for other people.
So, we need something else.
So, our idea is to just go beyond limitation,
think about how you should do that in
this predictable online learning settings and later on we
have designed some reduction based algorithm
such that we can leverage
the two that we already have for the adversarial settings,
so that we don't have to reinvent the views.
So now, we can have something that's going to work
robustly and going to work for most other people.
We call this algorithm or this framework PicCoLO.
It stands for Predictor-Corrector Policy Optimization.
It's a general reduction technique
for solving predictable online learning problems.
What it means is that it's
a problem where the cost function isn't linear,
and maybe the decision says convex.
The way it works is that,
it's going to convert let's say,
number n iterations, of a predictable online problems in here,
where the last function is specified by
some vector gn because that's a linear loss,
and it's going to convert that into a new adversarial problems,
and then just apply
the technique that we know on that new problem.
I will show that it actually works optimally.
The way we do that is, let's say we have some predictable vector,
coming out of [inaudible] gradient,
coming out of our predictive model that
I described earlier, right.
Just add there, and subtract that,
and you have this equality.
To simplify writing, let's define
the difference as an error, right.
Now, here's a trick.
You just add a new decision variable let's say CoPi
n hat adding here, and subtracting there.
What we end up having is that, on the left-hand side,
we will have a new adversarial problem,
which has two times more iterations.
The sequence was [inaudible].
You make decision Pi n hat before seeing gn hat and you get
gn hat and then you make decision Pi
n and then you see the new error en.
Because in this problem,
qn hat and en are unpredictable at all,
they are adversarial because you already subtract
all the predictable information that you
have from your predictive models.
So this is an adversarial problem.
Maybe the optimal weight to solve that,
you just apply the optimal area for that, right.
But would that give it optimal regret?
You have to ensure another thing,
is that the right-hand side.
The right-hand side if you look at that,
you will see that Pi n is actually made
after you reveal gn hat, right?
So, it's actually later on.
Which means that the blue box is actually negative.
So, what convergent means is that,
for a particular linear problem,
you can convert that into a new problem,
you apply adversarial technique to
that and if you show that the blue box is negative enough,
then you will have already convergence in terms of average regret.
This [inaudible] how picColO works.
PicColO, just apply a base algorithm for adversarial setting for
this new adversarial problem with two times more iterations.
We're going to show that this idea,
although it sounds very trivial,
has actually worked as optimally as a specialized version.
So, you can actually apply just, defined these,
convert to this new problem apply let's say,
[inaudible] to solve this one.
You don't need to know anything about
those specialized algorithms.
Also, we show that this will work for
any base algorithm thus can be written in the form of
mirror descent of other regress [inaudible] and those are basically
the optimal choices that you can have
for adversarial linear problems.
We have talked about more [inaudible] underlying problems,
pretty [inaudible] but now we want to focus back to a policy optimization.
So in order to do that,
so we have already shown
that imitation learning can
be written as predictable online problems.
But now, we want to go for [inaudible] and that.
So here we're all going to show that
actually reinforcement learning can also be written
as a predictable online problem
by some proper choice of the loss function sequence.
So, if we can do that,
then we can apply that technique to
both imitation learning and RL, right?
The way we do that is that we're going to define a loss function,
analog function as like this.
So here is equal to the expectation
of the definite function with respect to the previous policy.
Understand state distribution of the current policy.
It's kind of a way to measure how
a policy compares with the previous policy that you have.
If you look at this loss function which
might look weird but it's actually quite familiar.
If you take the gradient of this loss function,
where it says the thing on the right-hand side.
The thing on the right-hand side is actually
a practical version of the actor-critic gradient.
If you recall that for
the theoretical version of the actor-critic gradients is defined
like this but the adverse function
is with respect to the current policy, not the previous one.
Right? But that theoretical version is
usually not the one we implement because if we were to
implement that we need to run two raw [inaudible] per
iteration so that the gradient be [inaudible] unbiased.
What we run in practice is that, in each iteration,
we have the advantage function or the value function of
our previous policy and we
run the current policy collect some data,
we compute the actor-critic gradient,
and when you compute that gradient of the current policies,
actually using the previous advantage function
or Q functional, or value function. Then after that,
we're going to use a set experience to
update the value function estimate, right.
The advantage function or value function that we use to compute,
to implement actor-critic algorithms is always one step behind,
and this exactly the gradient of
this loss function that would define for IL in this conversion.
We can actually show that the average performance
of this policy optimization is bounded by
the performance of the initial policy plots the regret
down and Epsilon for this new loss functions and loss sequence.
So here regret is defined
as the simple version or the loss function.
So this L, delta L n is equal to
the simple version of that kind of
actor-critic loss function that we have,
and so here is a regret.
Here the Epsilon is the one we defined for imitation learning,
is like the minimum that you can
have for that sequence or loss function.
Suppose now we have a no regret algorithm,
which means that this regret is going to be
the sub-linear then the turn on
the left-hand index [inaudible] was going to be less than or [inaudible] one, right.
But now if this Epsilon is always more than negative or [inaudible] one.
Then this term will become negative one.
Which means that on average,
you're going to improve
roughly the same as number of iterations that you have.
But there are sometimes some digits we can discuss offline about,
let's say, how large the Epsilon actually is.
We see the problem of having
super large Epsilon in some worse case problems, right.
But this part has to be worked
out and then we can talk about some ideas about that later on,
but here let's assume that Epsilon can be
negative one in some nice region locally,
and this basically says that if you use
that practical actor-critic gradient.
It actually gives like a linear and not linear in that sense
but it will improve as a number of iteration that you perform.
Right. Now, we have imitation learning
and RL both of them to predictable online learning problems.
Now, it gives us a result of trying to
understand whether we can use that PicColO to learn those, right?
To give a more concrete example,
let us consider best algorithm which is
merely [inaudible] might be the one we are mostly familiar with.
What it does is that in here,
because we use policy optimization so that the direction will
be the practical actor-critic gradient that I talked
about or the gradient from
the imitation learning loss function
and here you have a [inaudible] divergence.
Which is regularization about how far did Pi closed with Pi n.
So if they are primitive divergence [inaudible]
square [inaudible] square norm then you will get gradient descent.
Basically, here you have to focus on two paths.
One's the gradient direction or the direction you want to
take and the other things is the regularization that you choose.
For now, we can think about the regularization basically is,
determine the step size or the problem.
If you look closer into this problem,
this update rule, it actually performs two-step rather than one.
You have to first choose a regularization first,
and the way you do that is you look into
the current gradient or vector that
you have and you choose some step size,
and after that, you use that step size to
update your policy along that gradient direction.
All right. So, if I'm to apply PicCoLO for policy optimization,
we need to know how to define
that gradient direction for the problem, right.
So, let's go back to the previous reduction.
So, reduction says that we can apply this mirror
descend on this new adversarial problem.
So, the gradient you will see is that the first gradient
will be the prediction gradient from your model.
In this case, the prediction gradient can come in
many different forms based on how this handles models,
and here are some common examples that you can now use.
So, you can, let's say you have a simulator.
If it's a dynamics model, you can compute
a simulated gradient as that gradient g and
Hat or another thing that you probably will
want to use in let's say in simulation or your problems,
is that you can actually use of policy gradients.
Let's say a gradient computing using symbols from other policies,
or maybe you can use gradient computing by a replay buffer.
So, this is actually what people use in practice,
I mean not in this way,
but just as a direct way to update policies.
But now, we're treating that as
another gradient not the exact one,
and now of course we can also learn UNL or
some other function approximated to
predict that gradient as well because,
we have a measure of the true gradient or we do interactions,
and then so we can actually learn that online or offline.
So, this is the first gradient.
The second one we need to do,
is we need to compute the error.
So, the only thing that's missing is the G n,
which is the one from the environment
and that one will be just the same
as the actor-critic gradients or
the material gradient that we have from alanine part.
So, now we have definitions of those.
Now, we can actually compare PicCoLO
with other approaches that we know about policy optimization.
So, let's do another free one.
So, the model free one what you do is that
you interrupt environmental current policy,
you get the gradient you care about,
and then you just apply the center middle design step.
That's how you can coalesce initial gradient design
or trust region processed image and so on.
So, you use that gradient,
you use that to decide your step size,
and then update your policy in the direction of the gradient.
This is the most common thing.
There's another approach to deport
data management which is a model-based one.
So, in here you interact with the environment,
but you never used the Gn to directly update the policy.
You use that experience to author your model,
and you model, it'll give you some other gradient.
For example, this describes approach of
using replayed buffering RL.
Right, you use that experience after
your model which is replay buffer,
and then now you use the replay
buffer gradient to update your policy.
You never actually use the gradient
from the environment directly and then,
you can also think about this as a simplified version
of the model-based RL in terms of the ultimate control approach,
where they use more iterations in the both side,
but here we are simplifying dying to one gradient step.
But it turns out that this reciprocation
returns either most of
the important structure and the
property of the model-based approach.
So, these are two common approaches.
All right. So, next one is Dyna.
Dyna is a very old algorithm proposed by Sudden,
and so what it does is basically doing
one model-based step and model-free step.
So, you call your model to
give you a gradient and other your policy,
and then you interact to get
a true gradient and under your policy.
You just treat the model-gradient
as exact one, and it's still the same thing.
For effectively doing twice iteration per area to interaction.
This wise maybe faster if the model is very well.
So, now it's PicCoLO.
So, PicCoLO has two parts: Recording the first part of
the prediction star and there is the [inaudible].
So, the main difference is that,
let's say comparing with Dyna this hybrid approach,
the main difference present in the prediction step,
we do not change the step size based
on the model gradient as in Dyna.
This are very important difference,
and the second important difference is that,
in a correctional step,
we are going to adjust our step size
based on that prediction arrow and then,
using that new step size to go along the arrow direction,
rather than the true gradient direction.
So, here we are going in the arrow and then here you're
doing the gradient on the left-hand side in Dyna,
and of course we can also use that experience to
opt their model, right.
So, what happens is that when you opt that,
when you choose step size and update direction in this way,
you will basically increase
your step size if your prediction error is small.
If your prediction is well, basically inside,
you can trust the model more,
so you can take a larger step size.
So, this is important because we are best interested in
steps size based on the accuracy of your model.
If you're produce is always well,
let's say like local linear problem,
you can predict very well, you can take larger steps,
and it seems like the intuition about why we want to use
arrows to choose step size rather than
the gradient size, another thing is that,
we're going to use the arrow to update
the policy and the main reason for that is that it's going
to when you're updating the arrow direction it can
remove the bias or
the prediction error that you have in a previous iteration,
although you are now using the [inaudible].
So, they will not exactly cancel out but let me just cancel
out good enough so they eventually won't have bias.
Any questions? How much time do I have?
>> Around two [inaudible] 10 more minutes.
>> Okay, yeah it's good. So, let's
try to visualize that a little bit.
So, let's say we have a Catlin and
was trying to go to you the butterfly.
So, in the predictor step it can take
the prediction gradient and it
goes at that direction, follow that.
In the correction step, you're going to
see the true gradient and see
the error and now it's going to adjust
the step size based on that prediction error,
I mean the error that you have,
and going to the arrow direction.
Now, I do again with prediction step
using the previous step size from
the previous correction step and going
to these prediction direction.
But because previously you incurred
an error so now it's going to travel
a smaller step size than the previous one which
is one for example. Do that again,
now you see another error and because for now this is
somehow lucky so the prediction error become small.
So, now when you have a smaller error,
it's going to take a larger step size.
So, it's going to take larger than one
step size to go through there,
then the data starts the error.
Now, but let suppose your model somehow is wrong,
it give you a very wrong direction
which doesn't lead you to the goal,
and so you're going to travel in
that direction using the steps that are
used though it's going to be nice
because the previous prediction error is small.
But in the next step, because we see
the true gradient which is going to direct back to
the goal and then now you see deadlock error,
and then you can just decrease their sigh and
also traveling that direction back,
and now you're going to use
that to apply to the next prediction step and so on.
So, eventually we can prove
the convergence in terms of
aggregate graph for all these approaches.
So, the best one that we have is
the Model-free approach, just a mirror descent.
So, convergence roughly proportional
to dimensional gradient also the variance of a gradient,
but they're going to be unbiased,
at the end of everything will converge to zero.
You have this model-based approach like DYNA as well.
So, here they are faster when your mother's accurate,
which means I might have a smaller variance in
model prediction and also you have a 2,000 iterations in Dyna.
But, the drawbell draws these approaches that
they will have a constant error En,
which is due to the prediction bias error.
So, at the end, every regret
will not converge to zero due to prediction error.
In PicCoLO, what happens is that,
the En is moved into the numerator.
So, at the end, depth hot will
convert to zero even though the prediction is wrong.
But now, you will see that the gradient, the prediction error,
plus the variance of prediction is
less than the size of the true gradient,
which means that your prediction
have a relative error less than one.
Then, this convergence rate it's going to
be faster than the model-free approach.
So what it means is that, PicColO can convert
any base algorithm into a new algorithm that can use
models and it's going to accelerate when
the prediction error is small.
But it's still going to be unbiased in
the sense of average regret even when the model is wrong.
So, experiments.
So, we use that to draft different best algorithm that we know.
That we know work for aisle.
So, we know ADAM is okay. Not very good but, okay.
Then we know, you can use Adaptive Natural Gradient Descent but,
with a step set aside in favor of ADAM,
and now we can also use TRPO.
For instance, family often your descent and they can be using RL.
Then we're going to compare how they
perform with different models.
So, we can compare with assimilating models,
assimilating gradients, and of policy gradient replay buffer.
Even with an Adversarial model,
and to see whether our previous kind
of about that being unbiased is true or not.
So, first we started Cartpole,
which is simple and it doesn't require too much time to run.
So, we run that with the best over
the ADAM and then we compare that with different models.
So, the gray lines are based algorithm.
They appear on the left and right hand clock.
So, it's actually moved
over the right hand side because there's
overlap together on the left hand side.
Another thing we want to compare is the red line.
Which is the one using the true dynamics as a predictive model.
When the dynamics is true but their model doesn't
have a zero prediction error in this sense,
but it's a meaningful direction.
So you can see that a red line,
if you have tradition that's reasonable,
is faster than the gray one.
Now let's compare with how
DYNA and PicColO responds to a very wrong model.
So, on the left hand side we have a model that
is computed by using a randomly initialized Neuro-dynamics.
So, that prediction doesn't give you much information.
All right. So, you see that,
in here the blue line will
converge to the optimal lines without much bias.
But at the end, the dynamic version,
the green one will fluctuate a lot.
So, that's a bias that we have in DYNA.
Now let's even go to the even worst scenario.
So, let's consider an Adversarial model for some reason.
So, the FSR model will give you a gradient,
which is the negative of the previous true gradient.
Ties are very large constant.
Yes. It's going to crash you,
and you can imagine that DYNA in here it completely fails.
It can never learn because you
have large error and it just crashed.
Right. But here Piccolo converges,
but is slower than the model-free approach.
Right. This corresponds to here.
So, if your prediction error is large,
you won't be faster but,
you will still converge but slower.
So, similarly, you have nice model,
you can run faster but I feel like Random model like
Adversarial model one it still converge but slower.
So, now let's look into
more complicated disagreements in the Cartpole.
But here we're comparing net of
gradient descent and happier space hours.
And those are the gray lines
on the left hand side and right hand side.
So, you see that we have different models.
We use the samples from
the less experience and also we have replay popper for like,
I think a 100 rules from the previous experience,
and I also have this model from the true dynamics.
It determines whether PicColO can improve,
because this somehow resemble model that we thought of.
Right. So, we see that when you pick out that,
you can run faster as in this environment.
We'll try that in other environments,
analyzing this walker3D, and the color lines.
They are colorful, so that they can read
faster and there are most long gray line.
Right. So, we have some preliminary source
about showing that we have to recall bumps.
Right. But those might not be tight enough for practical purpose.
But that give us a direction about why PicColO could work.
Right. Now in some experience and there's an open margin,
we showed that empirically,
that PicColO actually kind of
satisfied the theoretical over their want.
Right. So, obvious is
that we provide a reduction-n based framework.
That can convert any base algorithm
that you'll be using to a new algorithm.
That can use model information.
So, you can think PicColO is unbiased way for you to safely use.
Like models, like autopsy gradients,
and repay buffer, for typical examples.
Important parts, that's going to adapt the use though,
so it's going to be optimal to the prediction error that you have,
and eventually it's going to be unbiased. That's quite important.
So, in conclusion for today's talk,
we shot at policy optimization is predictable in some sense.
Because the loss function shares
that information from the past loss function.
So, some information can be inferred.
Especially in the form of a predictive model
of appertaining gradients of the future,
and this means that the previous approach,
the adversarial, treating of those problems,
it's going to be this optimal.
There's a need for us to develop
a new algorithm for the direction,
and here we show a particular algorithm
to use predictable information.
The PicColO one and it's actually a meta algorithm,
they can reuse previous approaches.
And then we also showed that,
if you consider those information,
you give us a tighter bound in analysis.
Right. But there are so many open questions,
when you just discovered this.
Right. So, this is nonetheless,
limited to positive optimization,
and also like in here,
I think the current version on PicColO
is not the base use of predictive models.
Let's say you are pretty much exactly correct
in the first conceptual algorithm.
You can solve the following one step but here you cannot.
Right. So, how do we actually better use of models,
so that they can actually cover even faster
than the current conversion that we have?
And also like in here PicColO,
none of us is focusing on certain regret.
Another idea is that we can move to dynamic data regrets,
and that will actually tighten the bounds that we have
to convert the regret bound to the performance bound.
It's similar to that that
two Epsilon that we talked about imitation learning.
Yes. So, this concludes my talk.
I want to thank my collaborators.
So we have Xinyan Yan and Nolan Wagener,
and Evangelos Theodora, from Computer Tech and was my advisor,
Nathan Ratliff, founder media.
Yes. So, any questions?
>> One or two questions.
The first one about the doc.
So, one of the reason
the predictable online learning problem
came up and then imitation learning setting,
was because they were constructing these phi
ends to get at the state distribution mismatch, right?
>> Yes.
>> Could we try to get at that street distribution mismatch
directly linked to the fundamental problem?
There was an expectation over state visitation under the Pi -
>> Yes.
>> -that we needed to get it end,
rather than constructing a bunch of
like surrogate pions to get at it?
>> Yes.
>> Can be instead that directly go at
the Odyssey mismatch between
B Pi behavior versus D Pi of the policy?
>> So, for example,
I think you cannot do that long examples.
But if you have a dynamics model,
internal simulator you can do that.
Because when you have that knowledge,
you can do simulations in some sense.
You are actually having a way to define capital F,
and then just by querying the oracle,
you can basically know why you were out
performed in the real environment without
really doing any interactions.
But if you only have
samples coming from different policy without
reconstructing a sense of model from them,
then eventually, I think you might be able to do something like,
average naming some sense with
errors so that you can approximate that.
But you won't be perfect.
But by me, they'll be good enough.
So, actually you can think that as
a predictive model, there is a just like,
the thing I talked about using off- policy gradients
and off- policy repaint buffer.
Has put the two models. Right. But if you just use that directly,
you have bias because that's not going to be perfect.
But that can actually be combined
side PicColO framework and then just to make safe use often.
Does that answer your question? Okay.
>> Okay. Anyone else?
Right. Well done.
>> Okay. Thank you.
-------------------------------------------
Body found Tuesday in Lumberton identified as Hania Aguilar - Duration: 2:27.
-------------------------------------------
Pet business booming in Korea as new services enter market - Duration: 2:45.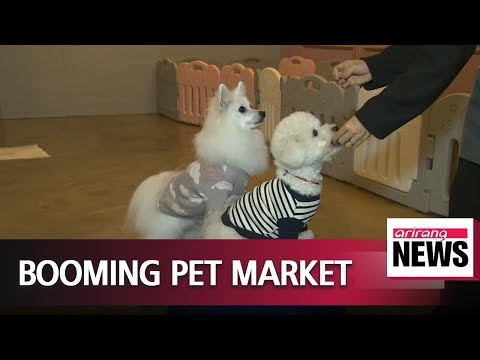
so more than 40% of people here in Korea take care of pets at home and looking to
tap into the multi-billion dollar market a wide range of services are popping up
catering to people who are willing to drop big money to make sure that their
furry friends are happy and healthy our sohn jung-in tells us more
every morning mr. Gabi feels a rush of excitement looking forward to playing
with his classmates he's arriving at a kindergarten for young pups when I was
in the office I modern with my dog through a security camera and it looked
alone and helpless he has become livelier since going to kindergarten
from early morn to evening to kindergarten daily schedule is packed
full of various activities that foster social skills physical strength and
relieve stress working women of all age groups are our major customers poppies
release their stress by playing with the other animals owned activities news work
is especially popular with the class news work is training for dogs to find
hidden stacks using their sense of smell because dogs released stress largely
through their sense of smell is a fun challenge customized activities aimed at
improving the dog's physical health are also offered dogs small medium sized
ones in particular often suffer from knee problems jumping or going under
hurdles and balance exercises help to strengthen their related muscles more
and more people see their past as companions or an extension of their
family as a result a bunch of new services including puppy kindergardens
have popped up it is hoped that such a services boost the well-being or past
and their owners miss Park is taking a cat to a vet the cash she's taking is a
taxi solely dedicated to packs both had an owner accountable and enjoyed the
ride though it is more than twice the price of a regular taxi more and more
people are making use of the service our services are mainly used by women of
all ages who either own a large pet or several animals when my cat got sick
last year taxi drivers refused to give us a ride
to the hospital and bus passengers looked annoyed a ride hailing service
for pets a salty issue with a multi-billion dollar market I suspected
this year and workers projected more unique and eye-catching services likely
to continue bursting onto the scene Ssangyong arirang news
-------------------------------------------
How To Ban Someone On Twitch As A Mod - Duration: 2:44.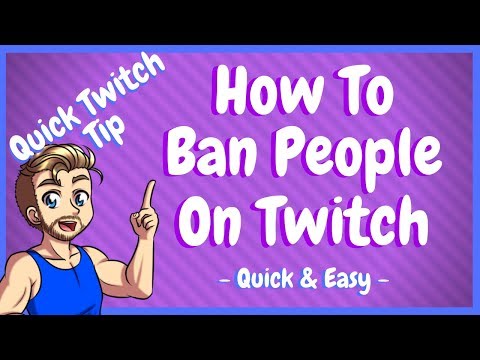
Need to know how to ban someone on twitch as a mod in this video I got you
covered so you can squash those trolls let's go!
Hey it's me it's wild coming at
you with another twitch quick tip video and if this is your first time here you
want to learn how to grow and improve your stream make sure you hit that big
red subscribe button and tick that bell so you know when my videos go live for
you now if you're a moderator or you're the actual broadcaster of your own
stream and you're having somebody that's doing a little too much spam or they're
putting too many links or they're being a little bit pushy or even just a
plain-out troll and not treating you nicely knowing how to ban someone as a
moderator or a broadcaster is something that you're gonna need to know so that
way you can run a smooth going stream and banning someone and unbanning
someone is something that's really easy to do so let me take you to the computer
and show you the exact commands or what you have to do to ban someone let's go
now that we're over at the computer there are two easy ways to ban somebody
from your channel as a moderator or as a broadcaster the simplest one being just
simply click on that users name and in the bottom left hand corner will be a
sign with a line through it this is the band command you simply just tick on it
and they will be banned from your chat the next easiest way to do is type in
/ban space put the user's name in there and hit enter once you enter they'll
instantly be banned from your chat if you accidently ban the wrong person all
you need to do is put in forward slash unban space and that user's name and
they will be unbanned and can type back in your chat now that you know how to ban
an unbanned people as a moderator or even as a broadcaster for streams on
Twitch don't be shy to actually throw down that ban hammer
just don't go too crazy give people a chance to say sorry so that way you can
try to grow and grab another new viewer hey if you guys like these videos and
you want me to do more twitch quick tip videos make sure you let me know in the
comments below and leave me a nice thumbs up
and if you want to keep up with me and my channel and all the art that I do
make sure you follow me on Twitter and Instagram at wild for games and if you
want to see me stream or you need any help with stream coaching on Twitch
youtube and mixer make sure you head on over to twitch at twitch.tv/wild4games
and hit that almighty subscribe button and fill up the stream
coaching form I can't wait to help you guys grow thank you so much for watching
this video and I will be back in my next twitch quick tip video coming up real
soon take care all peace
Không có nhận xét nào:
Đăng nhận xét